Yoshiki Masuyama
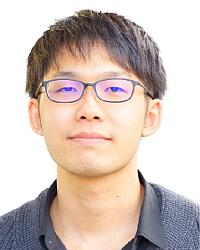
- Email:
-
Position:
Research / Technical Staff
Visiting Research Scientist -
Education:
Ph.D., Tokyo Metropolitan University, 2024 -
Research Areas:
External Links:
Yoshiki's Quick Links
-
Biography
Yoshiki's research interests focus on the integration of signal processing and machine learning technologies for efficient and robust audio processing. He has worked on a wide range of audio signal processing tasks, especially multichannel speech separation, robust automatic speech recognition, and multimodal learning. He is the recipient of the Best Student Paper Award at the IEEE Spoken Language Technology Workshop 2022.
-
Recent News & Events
-
NEWS MERL Researchers to Present 2 Conference and 11 Workshop Papers at NeurIPS 2024 Date: December 10, 2024 - December 15, 2024
Where: Advances in Neural Processing Systems (NeurIPS)
MERL Contacts: Petros T. Boufounos; Matthew Brand; Ankush Chakrabarty; Anoop Cherian; François Germain; Toshiaki Koike-Akino; Christopher R. Laughman; Jonathan Le Roux; Jing Liu; Suhas Lohit; Tim K. Marks; Yoshiki Masuyama; Kieran Parsons; Kuan-Chuan Peng; Diego Romeres; Pu (Perry) Wang; Ye Wang; Gordon Wichern
Research Areas: Artificial Intelligence, Communications, Computational Sensing, Computer Vision, Control, Data Analytics, Dynamical Systems, Machine Learning, Multi-Physical Modeling, Optimization, Robotics, Signal Processing, Speech & Audio, Human-Computer Interaction, Information SecurityBrief- MERL researchers will attend and present the following papers at the 2024 Advances in Neural Processing Systems (NeurIPS) Conference and Workshops.
1. "RETR: Multi-View Radar Detection Transformer for Indoor Perception" by Ryoma Yataka (Mitsubishi Electric), Adriano Cardace (Bologna University), Perry Wang (Mitsubishi Electric Research Laboratories), Petros Boufounos (Mitsubishi Electric Research Laboratories), Ryuhei Takahashi (Mitsubishi Electric). Main Conference. https://neurips.cc/virtual/2024/poster/95530
2. "Evaluating Large Vision-and-Language Models on Children's Mathematical Olympiads" by Anoop Cherian (Mitsubishi Electric Research Laboratories), Kuan-Chuan Peng (Mitsubishi Electric Research Laboratories), Suhas Lohit (Mitsubishi Electric Research Laboratories), Joanna Matthiesen (Math Kangaroo USA), Kevin Smith (Massachusetts Institute of Technology), Josh Tenenbaum (Massachusetts Institute of Technology). Main Conference, Datasets and Benchmarks track. https://neurips.cc/virtual/2024/poster/97639
3. "Probabilistic Forecasting for Building Energy Systems: Are Time-Series Foundation Models The Answer?" by Young-Jin Park (Massachusetts Institute of Technology), Jing Liu (Mitsubishi Electric Research Laboratories), François G Germain (Mitsubishi Electric Research Laboratories), Ye Wang (Mitsubishi Electric Research Laboratories), Toshiaki Koike-Akino (Mitsubishi Electric Research Laboratories), Gordon Wichern (Mitsubishi Electric Research Laboratories), Navid Azizan (Massachusetts Institute of Technology), Christopher R. Laughman (Mitsubishi Electric Research Laboratories), Ankush Chakrabarty (Mitsubishi Electric Research Laboratories). Time Series in the Age of Large Models Workshop.
4. "Forget to Flourish: Leveraging Model-Unlearning on Pretrained Language Models for Privacy Leakage" by Md Rafi Ur Rashid (Penn State University), Jing Liu (Mitsubishi Electric Research Laboratories), Toshiaki Koike-Akino (Mitsubishi Electric Research Laboratories), Shagufta Mehnaz (Penn State University), Ye Wang (Mitsubishi Electric Research Laboratories). Workshop on Red Teaming GenAI: What Can We Learn from Adversaries?
5. "Spatially-Aware Losses for Enhanced Neural Acoustic Fields" by Christopher Ick (New York University), Gordon Wichern (Mitsubishi Electric Research Laboratories), Yoshiki Masuyama (Mitsubishi Electric Research Laboratories), François G Germain (Mitsubishi Electric Research Laboratories), Jonathan Le Roux (Mitsubishi Electric Research Laboratories). Audio Imagination Workshop.
6. "FV-NeRV: Neural Compression for Free Viewpoint Videos" by Sorachi Kato (Osaka University), Takuya Fujihashi (Osaka University), Toshiaki Koike-Akino (Mitsubishi Electric Research Laboratories), Takashi Watanabe (Osaka University). Machine Learning and Compression Workshop.
7. "GPT Sonography: Hand Gesture Decoding from Forearm Ultrasound Images via VLM" by Keshav Bimbraw (Worcester Polytechnic Institute), Ye Wang (Mitsubishi Electric Research Laboratories), Jing Liu (Mitsubishi Electric Research Laboratories), Toshiaki Koike-Akino (Mitsubishi Electric Research Laboratories). AIM-FM: Advancements In Medical Foundation Models: Explainability, Robustness, Security, and Beyond Workshop.
8. "Smoothed Embeddings for Robust Language Models" by Hase Ryo (Mitsubishi Electric), Md Rafi Ur Rashid (Penn State University), Ashley Lewis (Ohio State University), Jing Liu (Mitsubishi Electric Research Laboratories), Toshiaki Koike-Akino (Mitsubishi Electric Research Laboratories), Kieran Parsons (Mitsubishi Electric Research Laboratories), Ye Wang (Mitsubishi Electric Research Laboratories). Safe Generative AI Workshop.
9. "Slaying the HyDRA: Parameter-Efficient Hyper Networks with Low-Displacement Rank Adaptation" by Xiangyu Chen (University of Kansas), Ye Wang (Mitsubishi Electric Research Laboratories), Matthew Brand (Mitsubishi Electric Research Laboratories), Pu Wang (Mitsubishi Electric Research Laboratories), Jing Liu (Mitsubishi Electric Research Laboratories), Toshiaki Koike-Akino (Mitsubishi Electric Research Laboratories). Workshop on Adaptive Foundation Models.
10. "Preference-based Multi-Objective Bayesian Optimization with Gradients" by Joshua Hang Sai Ip (University of California Berkeley), Ankush Chakrabarty (Mitsubishi Electric Research Laboratories), Ali Mesbah (University of California Berkeley), Diego Romeres (Mitsubishi Electric Research Laboratories). Workshop on Bayesian Decision-Making and Uncertainty. Lightning talk spotlight.
11. "TR-BEACON: Shedding Light on Efficient Behavior Discovery in High-Dimensions with Trust-Region-based Bayesian Novelty Search" by Wei-Ting Tang (Ohio State University), Ankush Chakrabarty (Mitsubishi Electric Research Laboratories), Joel A. Paulson (Ohio State University). Workshop on Bayesian Decision-Making and Uncertainty.
12. "MEL-PETs Joint-Context Attack for the NeurIPS 2024 LLM Privacy Challenge Red Team Track" by Ye Wang (Mitsubishi Electric Research Laboratories), Tsunato Nakai (Mitsubishi Electric), Jing Liu (Mitsubishi Electric Research Laboratories), Toshiaki Koike-Akino (Mitsubishi Electric Research Laboratories), Kento Oonishi (Mitsubishi Electric), Takuya Higashi (Mitsubishi Electric). LLM Privacy Challenge. Special Award for Practical Attack.
13. "MEL-PETs Defense for the NeurIPS 2024 LLM Privacy Challenge Blue Team Track" by Jing Liu (Mitsubishi Electric Research Laboratories), Ye Wang (Mitsubishi Electric Research Laboratories), Toshiaki Koike-Akino (Mitsubishi Electric Research Laboratories), Tsunato Nakai (Mitsubishi Electric), Kento Oonishi (Mitsubishi Electric), Takuya Higashi (Mitsubishi Electric). LLM Privacy Challenge. Won 3rd Place Award.
MERL members also contributed to the organization of the Multimodal Algorithmic Reasoning (MAR) Workshop (https://marworkshop.github.io/neurips24/). Organizers: Anoop Cherian (Mitsubishi Electric Research Laboratories), Kuan-Chuan Peng (Mitsubishi Electric Research Laboratories), Suhas Lohit (Mitsubishi Electric Research Laboratories), Honglu Zhou (Salesforce Research), Kevin Smith (Massachusetts Institute of Technology), Tim K. Marks (Mitsubishi Electric Research Laboratories), Juan Carlos Niebles (Salesforce AI Research), Petar Veličković (Google DeepMind).
- MERL researchers will attend and present the following papers at the 2024 Advances in Neural Processing Systems (NeurIPS) Conference and Workshops.
See All News & Events for Yoshiki -
-
Awards
-
AWARD MERL team wins the Listener Acoustic Personalisation (LAP) 2024 Challenge Date: August 29, 2024
Awarded to: Yoshiki Masuyama, Gordon Wichern, Francois G. Germain, Christopher Ick, and Jonathan Le Roux
MERL Contacts: François Germain; Jonathan Le Roux; Gordon Wichern; Yoshiki Masuyama
Research Areas: Artificial Intelligence, Machine Learning, Speech & AudioBrief- MERL's Speech & Audio team ranked 1st out of 7 teams in Task 2 of the 1st SONICOM Listener Acoustic Personalisation (LAP) Challenge, which focused on "Spatial upsampling for obtaining a high-spatial-resolution HRTF from a very low number of directions". The team was led by Yoshiki Masuyama, and also included Gordon Wichern, Francois Germain, MERL intern Christopher Ick, and Jonathan Le Roux.
The LAP Challenge workshop and award ceremony was hosted by the 32nd European Signal Processing Conference (EUSIPCO 24) on August 29, 2024 in Lyon, France. Yoshiki Masuyama presented the team's method, "Retrieval-Augmented Neural Field for HRTF Upsampling and Personalization", and received the award from Prof. Michele Geronazzo (University of Padova, IT, and Imperial College London, UK), Chair of the Challenge's Organizing Committee.
The LAP challenge aims to explore challenges in the field of personalized spatial audio, with the first edition focusing on the spatial upsampling and interpolation of head-related transfer functions (HRTFs). HRTFs with dense spatial grids are required for immersive audio experiences, but their recording is time-consuming. Although HRTF spatial upsampling has recently shown remarkable progress with approaches involving neural fields, HRTF estimation accuracy remains limited when upsampling from only a few measured directions, e.g., 3 or 5 measurements. The MERL team tackled this problem by proposing a retrieval-augmented neural field (RANF). RANF retrieves a subject whose HRTFs are close to those of the target subject at the measured directions from a library of subjects. The HRTF of the retrieved subject at the target direction is fed into the neural field in addition to the desired sound source direction. The team also developed a neural network architecture that can handle an arbitrary number of retrieved subjects, inspired by a multi-channel processing technique called transform-average-concatenate.
- MERL's Speech & Audio team ranked 1st out of 7 teams in Task 2 of the 1st SONICOM Listener Acoustic Personalisation (LAP) Challenge, which focused on "Spatial upsampling for obtaining a high-spatial-resolution HRTF from a very low number of directions". The team was led by Yoshiki Masuyama, and also included Gordon Wichern, Francois Germain, MERL intern Christopher Ick, and Jonathan Le Roux.
-
AWARD MERL team wins the Audio-Visual Speech Enhancement (AVSE) 2023 Challenge Date: December 16, 2023
Awarded to: Zexu Pan, Gordon Wichern, Yoshiki Masuyama, Francois Germain, Sameer Khurana, Chiori Hori, and Jonathan Le Roux
MERL Contacts: François Germain; Chiori Hori; Sameer Khurana; Jonathan Le Roux; Gordon Wichern; Yoshiki Masuyama
Research Areas: Artificial Intelligence, Machine Learning, Speech & AudioBrief- MERL's Speech & Audio team ranked 1st out of 12 teams in the 2nd COG-MHEAR Audio-Visual Speech Enhancement Challenge (AVSE). The team was led by Zexu Pan, and also included Gordon Wichern, Yoshiki Masuyama, Francois Germain, Sameer Khurana, Chiori Hori, and Jonathan Le Roux.
The AVSE challenge aims to design better speech enhancement systems by harnessing the visual aspects of speech (such as lip movements and gestures) in a manner similar to the brain’s multi-modal integration strategies. MERL’s system was a scenario-aware audio-visual TF-GridNet, that incorporates the face recording of a target speaker as a conditioning factor and also recognizes whether the predominant interference signal is speech or background noise. In addition to outperforming all competing systems in terms of objective metrics by a wide margin, in a listening test, MERL’s model achieved the best overall word intelligibility score of 84.54%, compared to 57.56% for the baseline and 80.41% for the next best team. The Fisher’s least significant difference (LSD) was 2.14%, indicating that our model offered statistically significant speech intelligibility improvements compared to all other systems.
- MERL's Speech & Audio team ranked 1st out of 12 teams in the 2nd COG-MHEAR Audio-Visual Speech Enhancement Challenge (AVSE). The team was led by Zexu Pan, and also included Gordon Wichern, Yoshiki Masuyama, Francois Germain, Sameer Khurana, Chiori Hori, and Jonathan Le Roux.
-
-
MERL Publications
- "Spatially-Aware Losses for Enhanced Neural Acoustic Fields", NeurIPS 2024 Audio Imagination Workshop, December 2024.BibTeX
- @inproceedings{Ick2024dec,
- author = {{Ick, Christopher and Wichern, Gordon and Masuyama, Yoshiki and Germain, François G and Le Roux, Jonathan}},
- title = {Spatially-Aware Losses for Enhanced Neural Acoustic Fields},
- booktitle = {NeurIPS 2024 Audio Imagination Workshop},
- year = 2024,
- month = dec
- }
, - "Spatially-Aware Losses for Enhanced Neural Acoustic Fields", Tech. Rep. TR2024-169, Mitsubishi Electric Research Laboratories, Cambridge, MA, December 2024.BibTeX TR2024-169 PDF
- @techreport{MERL_TR2024-169,
- author = {Ick, Christopher; Wichern, Gordon; Masuyama, Yoshiki; Germain, François G; Le Roux, Jonathan},
- title = {Spatially-Aware Losses for Enhanced Neural Acoustic Fields},
- institution = {MERL - Mitsubishi Electric Research Laboratories},
- address = {Cambridge, MA 02139},
- number = {TR2024-169},
- month = dec,
- year = 2024,
- url = {https://www.merl.com/publications/TR2024-169/}
- }
, - "NIIRF: Neural IIR Filter Field for HRTF Upsampling and Personalization", IEEE International Conference on Acoustics, Speech, and Signal Processing (ICASSP), DOI: 10.1109/ICASSP48485.2024.10448477, March 2024, pp. 1016-1020.BibTeX TR2024-026 PDF Software
- @inproceedings{Masuyama2024mar,
- author = {Masuyama, Yoshiki and Wichern, Gordon and Germain, François G and Pan, Zexu and Khurana, Sameer and Hori, Chiori and Le Roux, Jonathan},
- title = {NIIRF: Neural IIR Filter Field for HRTF Upsampling and Personalization},
- booktitle = {IEEE International Conference on Acoustics, Speech, and Signal Processing (ICASSP)},
- year = 2024,
- pages = {1016--1020},
- month = mar,
- doi = {10.1109/ICASSP48485.2024.10448477},
- url = {https://www.merl.com/publications/TR2024-026}
- }
, - "Scenario-Aware Audio-Visual TF-GridNet for Target Speech Extraction", IEEE Workshop on Automatic Speech Recognition and Understanding (ASRU), DOI: 10.1109/ASRU57964.2023.10389618, December 2023.BibTeX TR2023-152 PDF Video
- @inproceedings{Pan2023dec2,
- author = {Pan, Zexu and Wichern, Gordon and Masuyama, Yoshiki and Germain, François G and Khurana, Sameer and Hori, Chiori and Le Roux, Jonathan},
- title = {Scenario-Aware Audio-Visual TF-GridNet for Target Speech Extraction},
- booktitle = {IEEE Workshop on Automatic Speech Recognition and Understanding (ASRU)},
- year = 2023,
- month = dec,
- doi = {10.1109/ASRU57964.2023.10389618},
- isbn = {979-8-3503-0689-7},
- url = {https://www.merl.com/publications/TR2023-152}
- }
,
- "Spatially-Aware Losses for Enhanced Neural Acoustic Fields", NeurIPS 2024 Audio Imagination Workshop, December 2024.
-
Software & Data Downloads